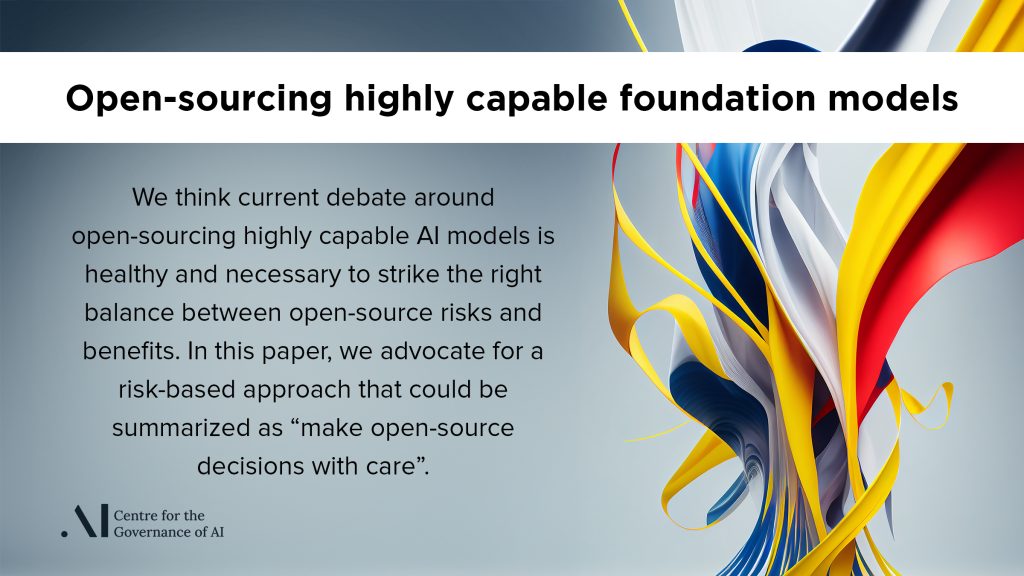
Open-Sourcing Highly Capable Foundation Models:
An Evaluation of Risks, Benefits, and Alternative Methods for Pursuing Open-Source Objectives.
September 2023
Seger, E., Dreksler, N., Moulange, R., Dardaman, E., Schuett, J., Wei, K., Winter, C., Arnold, M., Ó hÉigeartaigh, S., Korinek, A., Anderljung, M., Bucknall, B., Chan, A., Stafford, E., Koessler, L., Ovadya, A., Garfinkel, B., Bluemke. E., Aird, M., Levermore, P., Hazell, J., & Gupta, A.
Executive Summary
Recent decisions by AI developers to open-source foundation models have sparked debate over the prudence of open-sourcing increasingly capable AI systems. Open-sourcing in AI typically involves making model architecture and weights freely and publicly accessible for anyone to modify, study, build on, and use. On the one hand, this offers clear advantages including enabling external oversight, accelerating progress, and decentralizing AI control. On the other hand, it presents notable risks, such as allowing malicious actors to use AI models for harmful purposes without oversight and to disable model safeguards designed to prevent misuse.
This report attempts to clarify open-source terminology and to offer a thorough analysis of risks and benefits from open-sourcing AI. While open-sourcing has, to date, provided substantial net benefits for most software and AI development processes, we argue that for some highly capable models likely to emerge in the near future, the risks of open sourcing may outweigh the benefits.
There are three main factors underpinning this concern:
- Highly capable models have the potential for extreme risks. Of primary concern is diffusion of dangerous AI capabilities that could pose extreme risks—risk of significant physical harm or disruption to key societal functions. Malicious actors might apply highly capable systems, for instance, to help build new biological and chemical weapons, or to mount cyberattacks against critical infrastructures and institutions. We also consider other risks such as models helping malicious actors disseminate targeted misinformation at scale or to enact coercive population surveillance. Arguably, current AI capabilities do not yet surpass a critical threshold of capability for the most extreme risks. However, we are already seeing nascent dangerous capabilities emerge, and this trend is likely to continue as models become increasingly capable and as it becomes easier and requires less expertise and compute resources for users to deploy and fine-tune these models. (Section 3)
- Open-sourcing is helpful in addressing some risks, but could – overall – exacerbate the extreme risks that highly capable AI models may pose. While for traditional software, open-sourcing facilitates defensive activities to guard against misuse more so than it facilitates offensive misuse by malicious actors, the offense-defense balance is likely to skew more towards offense for increasingly capable foundation models. This is for a variety of reasons including: (i) Open-sourcing allows malicious actors to disable safeguards against misuse and to possibly introduce new dangerous capabilities via fine-tuning. (ii) Open-sourcing greatly increases attacker knowledge of possible exploits beyond what they would have been able to easily discover otherwise. (iii) Researching safety vulnerabilities is comparatively time consuming and resource intensive, and fixes are often neither straightforward nor easily implemented. (iv) It is more difficult to ensure improvements are implemented downstream, and flaws and safety issues are likely to perpetuate further due to the general use nature of the foundation models. (Section 3)
- There are alternative, less risky methods for pursuing open-source goals. There are a variety of strategies that might be employed to work towards the same goals as open-sourcing for highly capable foundation models but with less risk, albeit with their own shortcomings. These alternative methods include more structured model access options catered to specific research, auditing, and downstream development needs, as well as proactive efforts to organize secure collaborations, and to encourage and enable wider involvement in AI development, evaluation, and governance processes. (Section 4)
In light of these potential risks, limitations, and alternatives, we offer the following recommendations for developers, standards setting bodies, and governments. These recommendations are to help establish safe and responsible model sharing practices and to preserve open-source benefits where safe. They also summarize the paper’s main takeaways. (Section 5)
Recommendations:
- Developers and governments should recognize that some highly capable models will be too risky to open-source, at least initially. These models may become safe to open-source in the future as societal resilience to AI risk increases and improved safety mechanisms are developed.
- Decisions about open-sourcing highly capable foundation models should be informed by rigorous risk assessments. In addition to evaluating models for dangerous capabilities and immediate misuse applications, risk assessments must consider how a model might be fine-tuned or otherwise amended to facilitate misuse.
- Developers should consider alternatives to open-source release that capture some of the same distributive, democratic, and societal benefits, without creating as much risk. Some promising alternatives include gradual or “staged” model release, structured model access for researchers and auditors, and democratic oversight of AI development and governance decisions.
- Developers, standards setting bodies, and open-source communities should engage in collaborative and multi-stakeholder efforts to define fine-grained standards for when model components should be released. These standards should be based on an understanding of the risks posed by releasing different combinations of model components.
- Governments should exercise oversight of open-source AI models and enforce safety measures when stakes are sufficiently high. AI developers may not voluntarily adopt risk assessment and model sharing standards. Governments will need to enforce such measures through options such as liability law and regulation, for example via licensing requirements, fines, or penalties. They will also need to build the capacity to enforce such oversight mechanisms effectively. Immediate work is needed to evaluate the costs, consequences, and legal feasibility of various policy interventions and enforcement mechanisms we list.